Client Details
The customer has an advertisement bidding platform used by the largest retail company in the world. They process ~1BN messages per day.
Context
InfraCloud team built a global Kafka messaging platform on top of Mesos. The messages were processed by a big data pipeline.
Challenges
- The platform infrastructure should be cloud-agnostic, and it should be possible to deploy the whole platform at the click of a button with four 9s availability.
- Designing and implementing a Kafka based message queue platform to handle a high volume of messages for ad bidding platform (~ 1Bn per day)
- Some solution components were to be integrated with the big data pipeline; the big data solution itself was out of scope for this project.
Solution
- For container orchestration, we evaluated Kubernetes and DCOS Mesos. Based on maturity at that point and the requirement, we chose Mesos DCOS (2015).
- We had done POCs on Kafka and related management tools for visibility and monitoring for the message queue.
- We evaluated Ansible and SaltStack platform automation. We chose Saltstack as a solution.
- The prototype phase aimed to find the technologies fit for the use case by building and testing at a prototype scale.
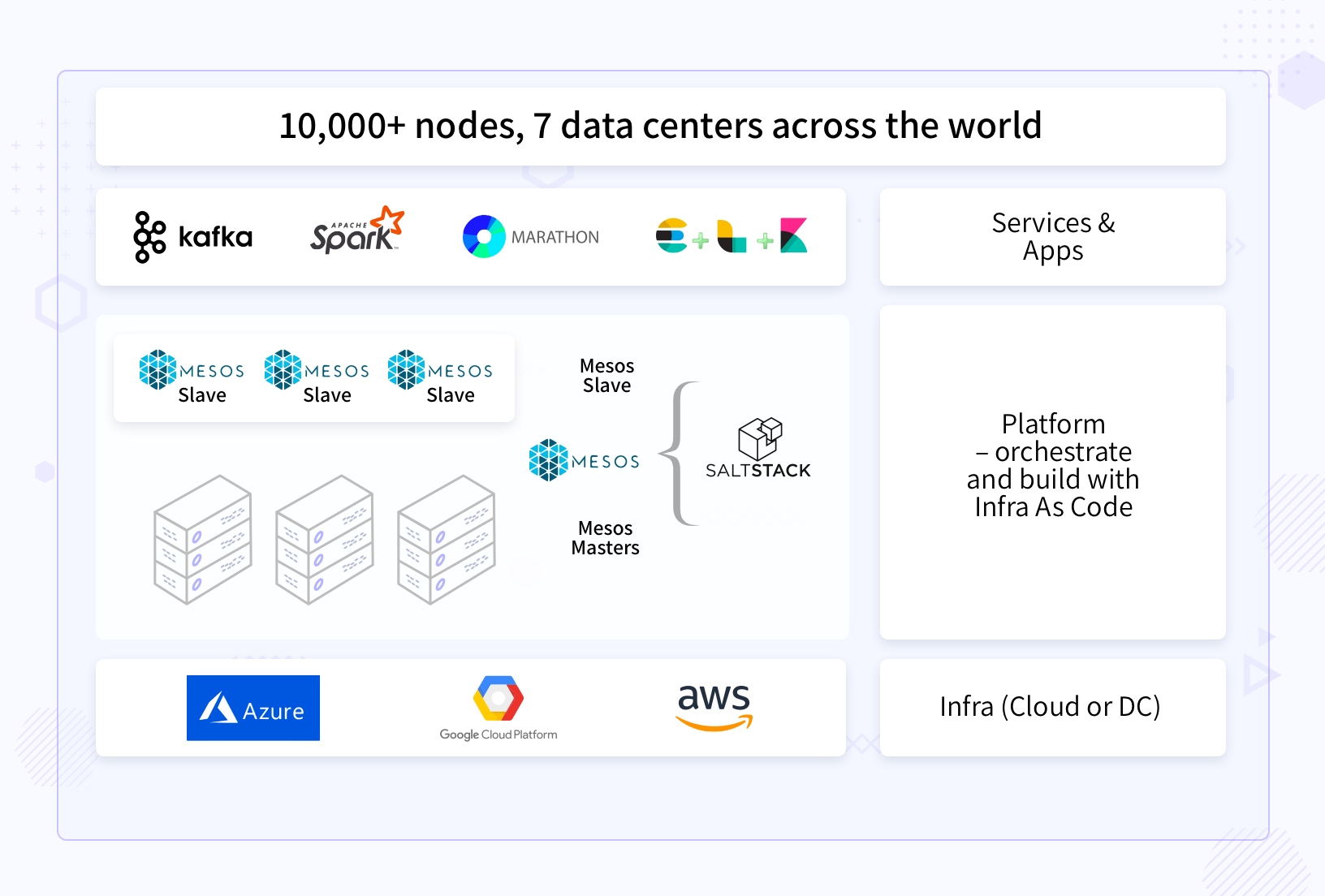
Solution - Infrastructure
- Cloud agnostic platform built with Saltstack & Mesos. Saltstack provisions the infra in any cloud and then sets up the Mesos cluster.
- On top of Mesos cluster services such as Kafka, ElasticSearch deployed using the DCOS framework. Application deployed as Dockerized containers using the Marathon framework.
- We replicated the Kafka cluster across availability zones (AZ) with the Kafka mirror.
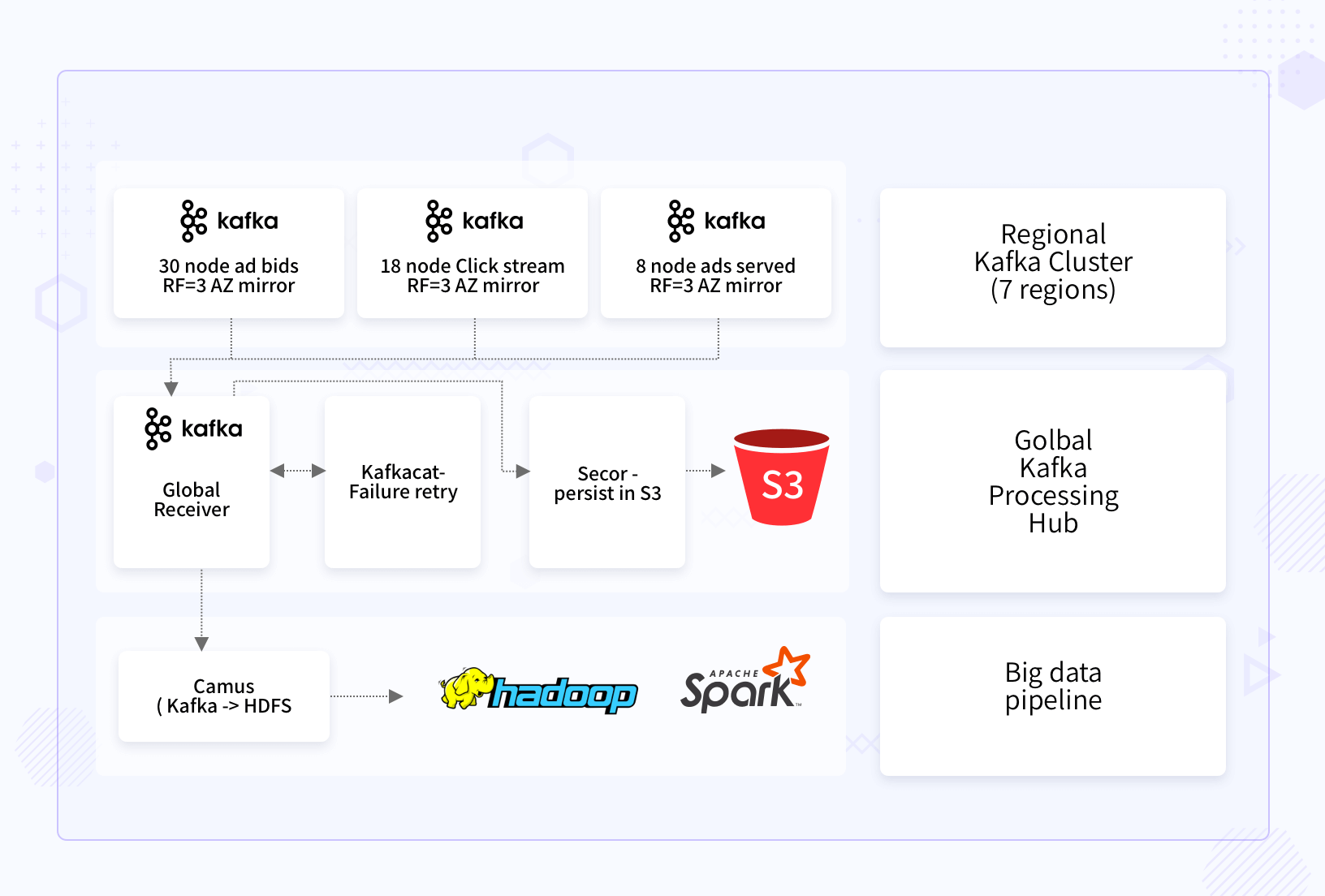
Solution - Messaging Platform
- We designed regional Kafka clusters for separating incoming messages based on scale and demand. Regional Kafka clusters were closed to the edge in 7 regions globally.
- Central Kafka cluster for finally sending filtered messages to the data pipeline
- A replication factor of 3 at the Kafka cluster level for high redundancy.
- S3 persistence for future replay and backup.
- Kafkacat for immediately replaying failed messages, if any.
- We used Camus to convert Kafka messages to HDFS and used by Hadoop batch jobs and Spark jobs.
Why InfraCloud?
- Our long history in programmable infrastructure space from VMs to containers give us an edge.
- We are one of cloud native technology thought leaders (speakers at various global CNCF conferences, authors, etc.).
- DevOps engineers who have pioneered DevOps at Fortune 500 companies.
- Our teams have worked from data center to deploying apps and across all phases of SDLC, bringing a holistic view of systems.